MDM as a Competitive Advantage in Organizations
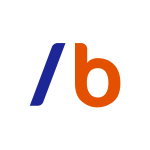
Maryury García
Cloud | Data & Analytics
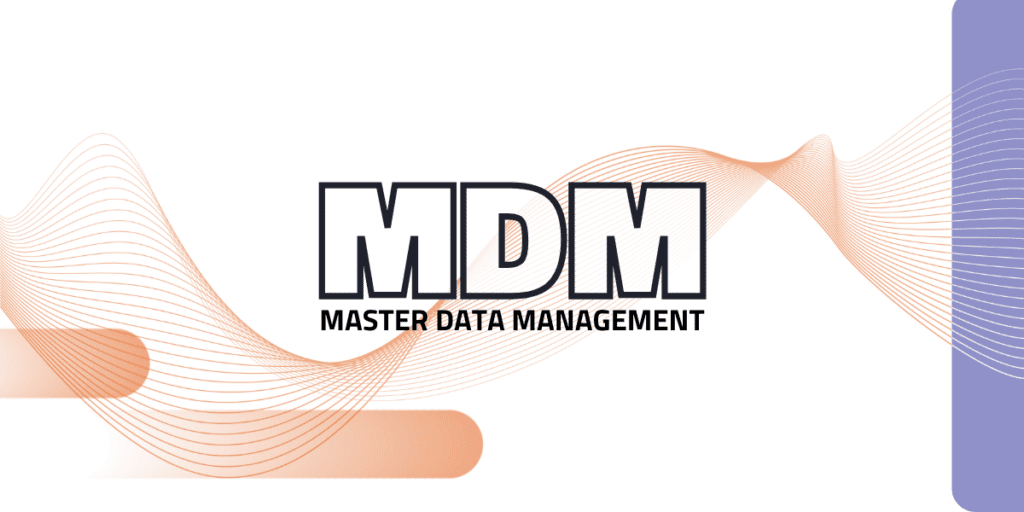
Just like natural resources, data acts as the driving fuel for innovation, decision-making, and value creation across various sectors. From large tech companies to small startups, digital transformation is empowering data to become the foundation that generates knowledge, optimizes efficiency, and offers personalized experiences to users.
Master Data Management (MDM) plays an essential role in providing a solid structure to ensure the integrity, quality, and consistency of data throughout the organization.
Despite this discipline existing since the mid-90s, some organizations have not fully adopted MDM. This could be due to various factors such as a lack of understanding of its benefits, cost, complexity, and/or maintenance.
According to a Gartner survey, the global MDM market was valued at $14.6 billion in 2022 and is expected to reach $24 billion by 2028, with a compound annual growth rate (CAGR) of 8.2%.
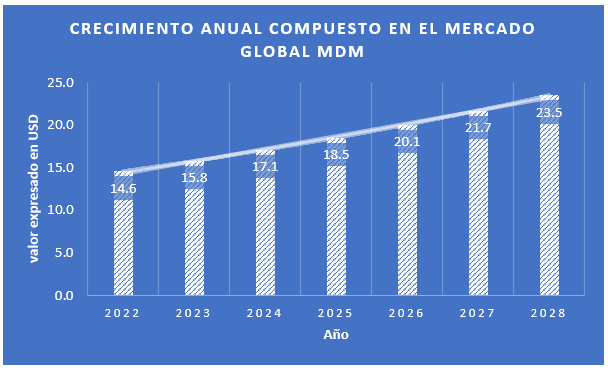
Before diving into the world of MDM, it is important to understand some relevant concepts. To manage master data, the first question we ask is: What is master data? Master data constitutes the set of shared, essential, and critical data for business execution. It has a lifecycle (validity period) and contains key information for the organization’s operation, such as customer data, product information, account numbers, and more.
Once defined, it is important to understand their characteristics, as master data is unique, persistent, and integral, with broad coverage, among other qualities. This is vital to ensure consistency and quality.
Therefore, it is essential to have an approach that considers both organizational aspects (identification of data owners, impacted users, matrices, etc.) as well as processes (related to policies, workflows, procedures, and mappings). Hence, our proposal at Bluetab on this approach is summarized in each of these dimensions.
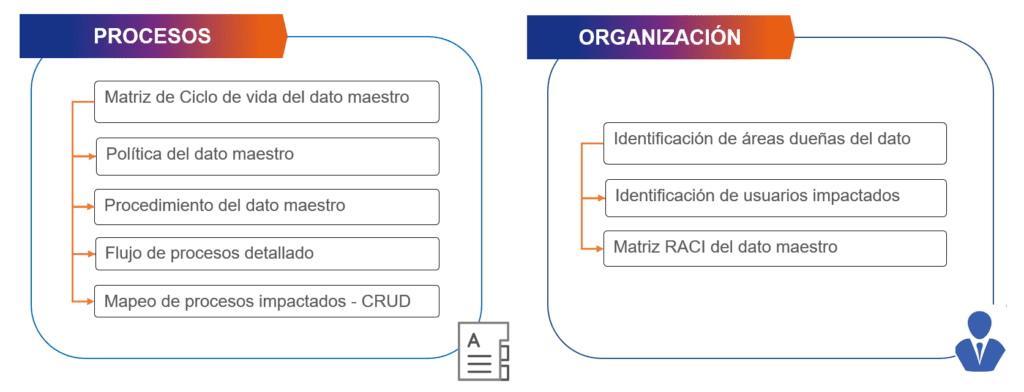
Another aspect to consider from our experience with master data, which is key to starting an organizational implementation, is understanding its “lifecycle.” This includes:
- The business areas inputting the master data (referring to the areas that will consume the information).
- The processes associated with the master data (that create, block, report, update the master data attributes—in other words, the treatment that the master data will undergo).
- The areas outputting the master data (referring to the areas that ultimately maintain the master data).
- All of this is intertwined with the data owners and supported by associated policies, procedures, and documentation.
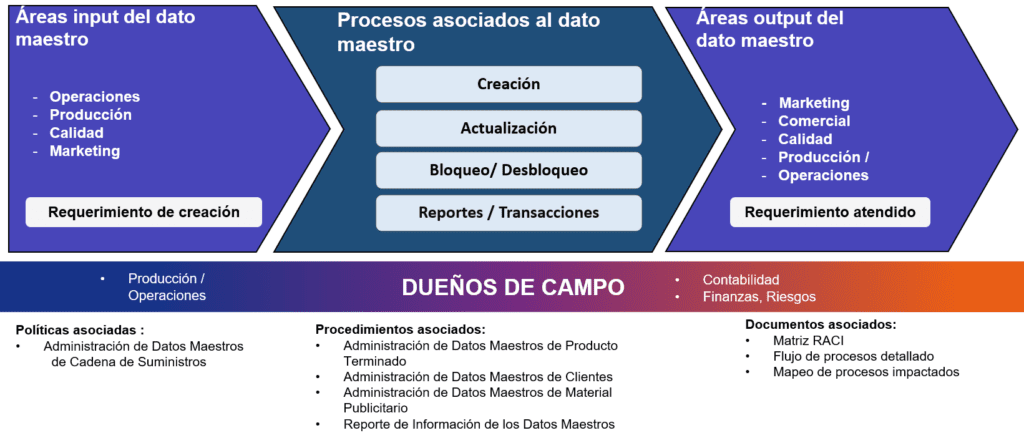
Master Data Management (MDM) is a “discipline,” and why? Because it brings together a set of knowledge, policies, practices, processes, and technologies (referred to as a technological tool to collect, store, manage, and analyze master data). This allows us to conclude that it is much more than just a tool.
Below, we provide some examples that will help to better understand the contribution of proper master data management in various sectors:
- Retail Sector: Retail companies, for example, a bakery, would use MDM to manage master data for product catalogs, customers, suppliers, employees, recipes, inventory, and locations. This creates a detailed customer profile to ensure a consistent and personalized shopping experience across all sales channels.
- Financial Sector: Financial institutions could manage customer data, accounts, financial products, pricing, availability, historical transactions, and credit information. This helps improve the accuracy and security of financial transactions and operations, as well as verify customer identities before opening an account.
- Healthcare Sector: In healthcare, the most important data is used to manage patient data, procedure data, diagnostic data, imaging data, medical facilities, and medications, ensuring the integrity and privacy of confidential information. For example, a hospital can use MDM to generate an EMR (Electronic Medical Record) for each patient.
- Telecommunications Sector: In telecommunications, companies use MDM to manage master data for their devices, services, suppliers, customers, and billing.
In Master Data Management, the following fundamental operations are performed: data cleaning, which removes duplicates; data enrichment, which ensures complete records; and the establishment of a single source of truth. The time it may take depends on the state of the organization’s records and its business objectives. Below, we can visualize the tasks that are carried out:
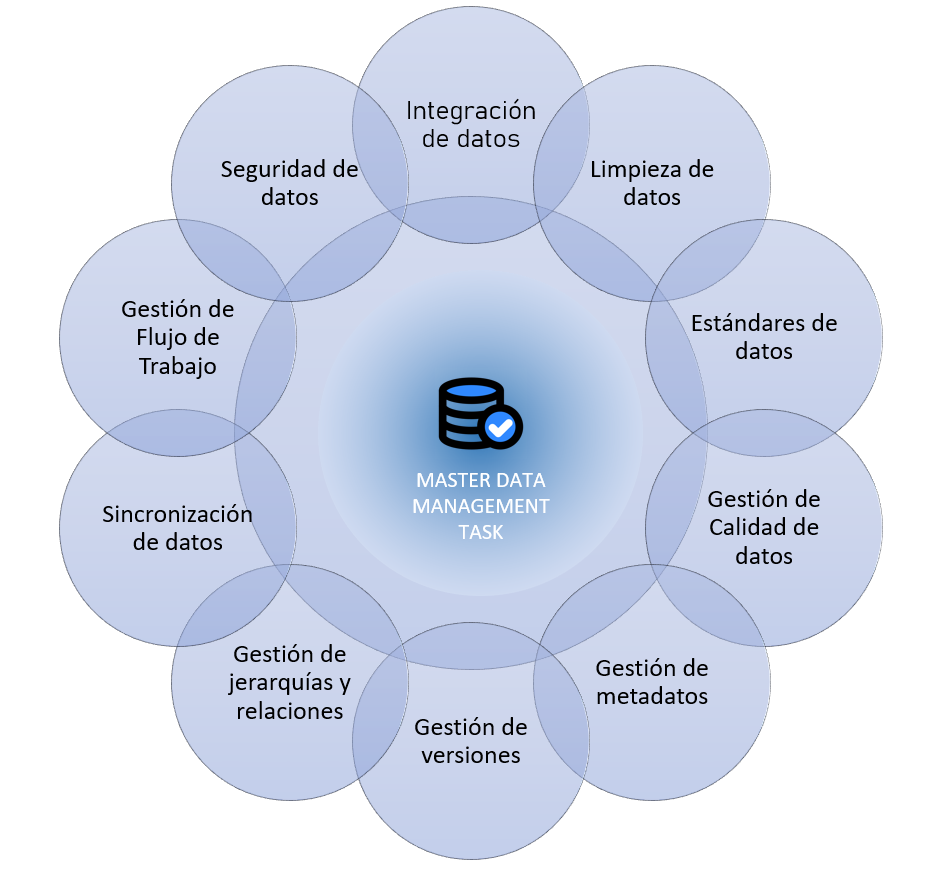
Now that we have a clearer concept, it’s important to keep in mind that the strategy for managing master data is to keep it organized: up-to-date, accurate, non-redundant, consistent, and integral.
What benefits does implementing an MDM provide?
- Data Quality and Consistency: Improves the quality of master data by eliminating duplicates and correcting errors, ensuring the integrity of information throughout the organization.
- Efficiency and Resource Savings: Saves time and resources by automating tasks of data cleaning, enrichment, and updating, freeing up staff for more strategic tasks.
- Informed Decision-Making: Allows the identification of patterns and trends from reliable data, driving strategic and timely decision-making.
- Enhanced Customer Experience: Improves the customer experience by providing a 360-degree view of the customer, enabling more personalized and relevant interactions.
- At Bluetab, we have helped clients from various industries with their master data strategy, from the definition, analysis, and design of the architecture to the implementation of an integrated solution. From this experience, we share these 5 steps to help you start managing master data:
List Your Objectives and Define a Scope
First, identify which data entities are of commercial priority within the organization. Once identified, evaluate the number of sources, definitions, exceptions, and volumes that the entities have.
Define the Data You Will Use
Which part of the data is important for decision-making? It could simply be all or several fields of the record to fill in, such as name, address, and phone number. Get support from governance personnel for the definition.
Establish Processes and Owners
Who will be responsible for having the rights to modify or create the data? For what and how will this data be used to reinforce or enhance the business? Once these questions are formulated, it is important to have a process for how the information will be handled from the master data registration to its final sharing (users or applications).
Seek Scalability
Once you have defined the processes, try to ensure they can be integrated with future changes. Take the time to define your processes and avoid making drastic changes in the future.
Find the Right Data Architecture, Don’t Take Shortcuts
Once the previous steps are defined and generated, it’s time to approach your Big Data & Analytics strategic partner to ensure these definitions are compatible within the system or databases that house your company’s information.
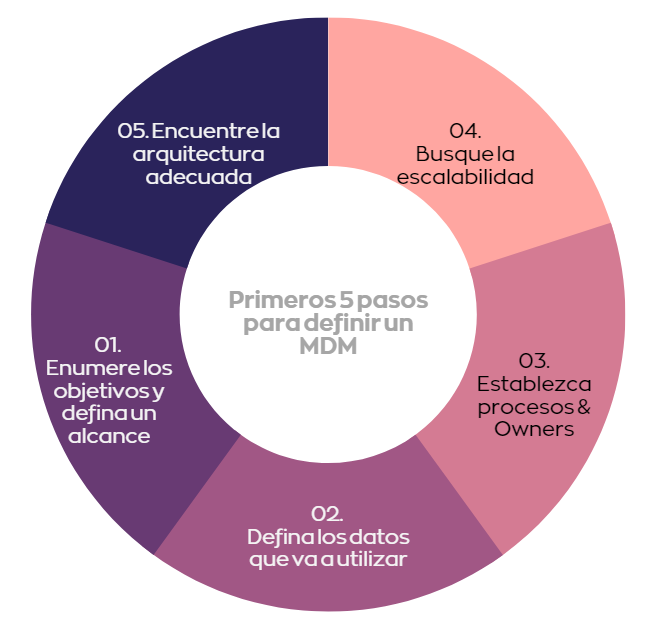
Final Considerations
Based on our experience, we suggest considering the following aspects when assessing/defining the process for each domain in master data management, subject to the project scope:
- Management of Routes:
- Consider how the owner of the creation of master data registers it (automatically and eliminating manual data entry from any other application) and how any current process of an area/person centralizes the information from other areas involved in the master data manually (emails, calls, Excel sheets, etc.). This should be automated in a workflow.
- Alerts & Notifications:
- It is recommended to establish deadlines for the completeness of the data for each area and the responsible party updating a master data.
- The time required to complete each data entry should be agreed upon among all involved areas, and alerts should be configured to communicate the updated master data.
- Blocking and Discontinuation Processes:
- A viable alternative is to make these changes operationally and then communicate them to the MDM through replication.
- Integration:
- Evaluate the possibility of integrating with third parties to automate the registration process for clients, suppliers, etc., and avoid manual entry: RENIEC, SUNAT, Google (coordinates X, Y, Z), or other agents, evaluating suitability for the business.
- Incorporation of Third Parties:
- Consider the incorporation of clients and suppliers at the start of the master data creation flows and at the points of updating.

In summary, master data is the most important common data for an organization and serves as the foundation for many day-to-day processes at the enterprise level. Master data management helps ensure that data is up-to-date, accurate, non-redundant, consistent, integral, and properly shared, providing tangible benefits in data quality, operational efficiency, informed decision-making, and customer experience. This contributes to the success and competitiveness of the organization in an increasingly data-driven digital environment.
If you found this article interesting, we appreciate you sharing it. At Bluetab, we look forward to hearing about the challenges and needs you have in your organization regarding master and reference data.
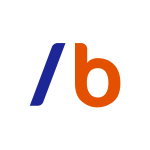
Maryury García
Cloud | Data & Analytics